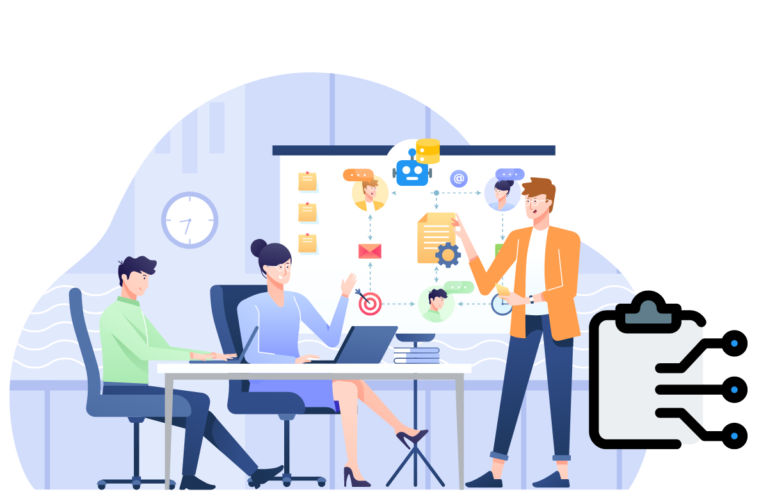
Artificial Frustration: The Top Hurdles in AI/ML Project Management
The AI Project Tightrope
Data Quality and Quantity
One of the primary challenges in AI project management is data quality and quantity. The quality and quantity of data play a significant role in the success of machine learning projects. The algorithms used in machine learning rely heavily on the data fed into them. If the data is incomplete and of good quality, it can lead to accurate results.
For example, a machine learning model was used in the retail industry to predict the demand for a particular product. However, the model was fed with limited time frame data, single dimensional, not including all internal and external key influencing factors, so the predictions were not accurate. A traditional business analytics-based approach was used as it was a project team that had limited expertise. This led to overstocking of the product, ultimately resulting in a significant loss for the company.
Ensuring that the data fed into the machine learning algorithm is accurate and complete is essential to avoid such scenarios. It is also important to have enough data to train the algorithm effectively. According to a report by Gartner, by 2022, 85% of AI projects will fail due to the lack of high-quality data.
Lack of Expertise
Another significant hurdle in AI project management is the need for more expertise. AI project management requires a specific set of skills that only some possess. It is important to have people on the team with experience in data science, machine learning, and AI.
In the healthcare industry, a machine learning project was initiated to predict the risk of heart disease in patients. However, the team needed to gain data science expertise, leading to a flawed algorithm. The model was not accurate, so it failed to predict the risk of heart disease accurately.
To avoid such scenarios, it is essential to have a team with diverse skill sets. According to a report by the World Economic Forum, by 2025, the demand for data analysts and scientists will increase by 4.4 million globally.
Integration with Existing Systems
Integrating AI projects with existing systems is another significant challenge. Most organizations have legacy systems that are different from modern AI systems. Integrating AI projects with these systems can be a daunting task, and it requires a significant amount of time and effort.
In the finance industry, a machine learning project was initiated to detect fraud in credit card transactions. However, the existing systems were not compatible with the new AI system. This led to delays in integrating the new system with the existing one, and as a result, it took much longer than anticipated to complete the project.
To avoid such scenarios, it is essential to have a clear understanding of the existing systems and their compatibility with modern AI systems. According to a report by Accenture, 85% of executives believe that integrating AI into their organizations is challenging.
Regulatory Compliance
Regulatory compliance can be a real buzzkill for AI and machine learning projects. It’s like having a hall monitor at a party, always watching over your shoulder and threatening to ruin all the fun. But the truth is, compliance is a necessary evil that must be dealt with if you want your AI project to succeed.
Let’s take a look at some examples of how regulatory compliance can be a challenge in various industries. A machine learning project was initiated in the retail industry to analyze customer data and personalize marketing campaigns. However, the project had to comply with data protection laws, making collecting and using customer data challenging. As a result, the project had to be modified to comply with the regulations, which delayed the implementation and reduced the effectiveness of the project.
A machine learning project was initiated in the manufacturing industry to optimize the production process. However, the project involved using personal data, which had to comply with data protection laws. The team had to implement additional security measures to protect the data, which added complexity and cost to the project.
In the finance industry, a machine learning project was initiated to detect fraud in credit card transactions. However, the project had to comply with strict regulations on data privacy and security. The team had to implement additional measures to ensure the data was secure, which added time and cost to the project.
According to a report by Deloitte, 76% of executives believe that regulatory compliance is the biggest barrier to adopting AI and machine learning projects. However, compliance is an obstacle that can be addressed. It’s essential to ensure that the project complies with all the relevant regulations to avoid fines, legal issues, and damage to the company’s reputation.
In conclusion, regulatory compliance can be a pain in the neck, but it’s a necessary evil to deal with if you want your AI and machine learning projects to succeed. Compliance is not something to be taken lightly, and it’s essential to ensure that the project complies with all the relevant regulations to avoid any legal issues or fines. So, keep the hall monitor happy and ensure your project is compliant from the start.